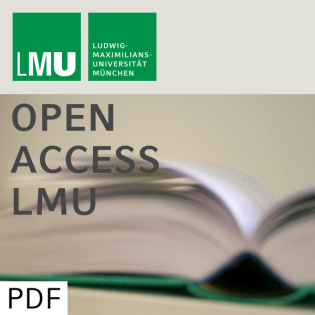
DTI denoising for data with low signal to noise ratios
Beschreibung
vor 19 Jahren
Low signal to noise ratio (SNR) experiments in diffusion tensor
imaging (DTI) give key information about tracking and anisotropy,
e. g., by measurements with small voxel sizes or with high b
values. However, due to the complicated and dominating impact of
thermal noise such data are still seldom analysed. In this paper
Monte Carlo simulations are presented which investigate the
distributions of noise for different DTI variables in low SNR
situations. Based on this study a strategy for the application of
spatial smoothing is derived. Optimal prerequisites for spatial
filters are unbiased, bell shaped distributions with uniform
variance, but, only few variables have a statistics close to that.
To construct a convenient filter a chain of nonlinear Gaussian
filters is adapted to peculiarities of DTI and a bias correction is
introduced. This edge preserving three dimensional filter is then
validated via a quasi realistic model. Further, it is shown that
for small sample sizes the filter is as effective as a maximum
likelihood estimator and produces reliable results down to a local
SNR of approximately 1. The filter is finally applied to very
recent data with isotropic voxels of the size 1×1×1mm^3 which
corresponds to a spatially mean SNR of 2.5. This application
demonstrates the statistical robustness of the filter method.
Though the Rician noise model is only approximately realized in the
data, the gain of information by spatial smoothing is considerable.
imaging (DTI) give key information about tracking and anisotropy,
e. g., by measurements with small voxel sizes or with high b
values. However, due to the complicated and dominating impact of
thermal noise such data are still seldom analysed. In this paper
Monte Carlo simulations are presented which investigate the
distributions of noise for different DTI variables in low SNR
situations. Based on this study a strategy for the application of
spatial smoothing is derived. Optimal prerequisites for spatial
filters are unbiased, bell shaped distributions with uniform
variance, but, only few variables have a statistics close to that.
To construct a convenient filter a chain of nonlinear Gaussian
filters is adapted to peculiarities of DTI and a bias correction is
introduced. This edge preserving three dimensional filter is then
validated via a quasi realistic model. Further, it is shown that
for small sample sizes the filter is as effective as a maximum
likelihood estimator and produces reliable results down to a local
SNR of approximately 1. The filter is finally applied to very
recent data with isotropic voxels of the size 1×1×1mm^3 which
corresponds to a spatially mean SNR of 2.5. This application
demonstrates the statistical robustness of the filter method.
Though the Rician noise model is only approximately realized in the
data, the gain of information by spatial smoothing is considerable.
Weitere Episoden



vor 11 Jahren
Kommentare (0)