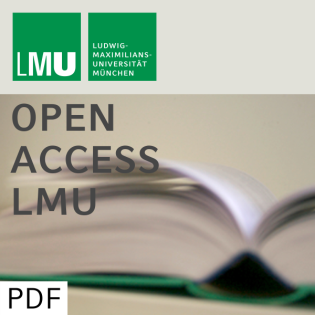
Variable selection with Random Forests for missing data
Beschreibung
vor 11 Jahren
Variable selection has been suggested for Random Forests to improve
their efficiency of data prediction and interpretation. However,
its basic element, i.e. variable importance measures, can not be
computed straightforward when there is missing data. Therefore an
extensive simulation study has been conducted to explore possible
solutions, i.e. multiple imputation, complete case analysis and a
newly suggested importance measure for several missing data
generating processes. The ability to distinguish relevant from
non-relevant variables has been investigated for these procedures
in combination with two popular variable selection methods.
Findings and recommendations: Complete case analysis should not be
applied as it lead to inaccurate variable selection and models with
the worst prediction accuracy. Multiple imputation is a good means
to select variables that would be of relevance in fully observed
data. It produced the best prediction accuracy. By contrast, the
application of the new importance measure causes a selection of
variables that reflects the actual data situation, i.e. that takes
the occurrence of missing values into account. It's error was only
negligible worse compared to imputation.
their efficiency of data prediction and interpretation. However,
its basic element, i.e. variable importance measures, can not be
computed straightforward when there is missing data. Therefore an
extensive simulation study has been conducted to explore possible
solutions, i.e. multiple imputation, complete case analysis and a
newly suggested importance measure for several missing data
generating processes. The ability to distinguish relevant from
non-relevant variables has been investigated for these procedures
in combination with two popular variable selection methods.
Findings and recommendations: Complete case analysis should not be
applied as it lead to inaccurate variable selection and models with
the worst prediction accuracy. Multiple imputation is a good means
to select variables that would be of relevance in fully observed
data. It produced the best prediction accuracy. By contrast, the
application of the new importance measure causes a selection of
variables that reflects the actual data situation, i.e. that takes
the occurrence of missing values into account. It's error was only
negligible worse compared to imputation.
Weitere Episoden


Kommentare (0)