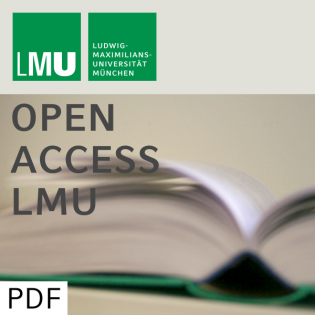
Propriety of Posteriors in Structured Additive Regression Models: Theory and Empirical Evidence
Beschreibung
vor 18 Jahren
Structured additive regression comprises many semiparametric
regression models such as generalized additive (mixed) models,
geoadditive models, and hazard regression models within a unified
framework. In a Bayesian formulation, nonparametric functions,
spatial effects and further model components are specified in terms
of multivariate Gaussian priors for high-dimensional vectors of
regression coefficients. For several model terms, such as penalised
splines or Markov random fields, these Gaussian prior distributions
involve rank-deficient precision matrices, yielding partially
improper priors. Moreover, hyperpriors for the variances
(corresponding to inverse smoothing parameters) may also be
specified as improper, e.g. corresponding to Jeffery's prior or a
flat prior for the standard deviation. Hence, propriety of the
joint posterior is a crucial issue for full Bayesian inference in
particular if based on Markov chain Monte Carlo simulations. We
establish theoretical results providing sufficient (and sometimes
necessary) conditions for propriety and provide empirical evidence
through several accompanying simulation studies.
regression models such as generalized additive (mixed) models,
geoadditive models, and hazard regression models within a unified
framework. In a Bayesian formulation, nonparametric functions,
spatial effects and further model components are specified in terms
of multivariate Gaussian priors for high-dimensional vectors of
regression coefficients. For several model terms, such as penalised
splines or Markov random fields, these Gaussian prior distributions
involve rank-deficient precision matrices, yielding partially
improper priors. Moreover, hyperpriors for the variances
(corresponding to inverse smoothing parameters) may also be
specified as improper, e.g. corresponding to Jeffery's prior or a
flat prior for the standard deviation. Hence, propriety of the
joint posterior is a crucial issue for full Bayesian inference in
particular if based on Markov chain Monte Carlo simulations. We
establish theoretical results providing sufficient (and sometimes
necessary) conditions for propriety and provide empirical evidence
through several accompanying simulation studies.
Weitere Episoden



vor 11 Jahren
Kommentare (0)