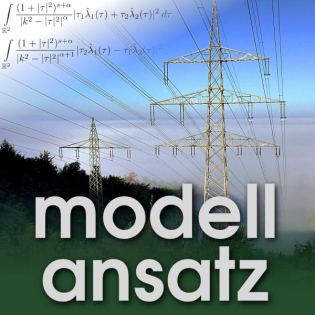
Podcast
Podcaster
Beschreibung
vor 5 Jahren
Gudrun Talks to Sema Coşkun who at the moment of the conversation
in 2018 is a Post Doc researcher at the University Kaiserslautern
in the group of financial mathematics. She constructs models for
the behaviour of energy markets.
In short the conversation covers the questions
How are classical markets modelled?
In which way are energy markets different and need new ideas?
The seminal work of Black and Scholes (1973) established the
modern financial theory. In a Black-Scholes setting, it is
assumed that the stock price follows a Geometric Brownian Motion
with a constant drift and constant volatility. The stochastic
differential equation for the stock price process has an explicit
solution. Therefore, it is possible to obtain the price of a
European call option in a closed-form formula. Nevertheless,
there exist drawbacks of the Black-Scholes assumptions. The most
criticized aspect is the constant volatility assumption. It is
considered an oversimplification. Several improved models have
been introduced to overcome those drawbacks. One significant
example of such new models is the Heston stochastic volatility
model (Heston, 1993). In this model, volatility is indirectly
modeled by a separate mean reverting stochastic process, namely.
the Cox-Ingersoll-Ross (CIR) process. The CIR process captures
the dynamics of the volatility process well. However, it is not
easy to obtain option prices in the Heston model since the model
has more complicated dynamics compared to the Black-Scholes
model.
In financial mathematics, one can use several methods to deal
with these problems. In general, various stochastic processes are
used to model the behavior of financial phenomena. One can then
employ purely stochastic approaches by using the tools from
stochastic calculus or probabilistic approaches by using the
tools from probability theory. On the other hand, it is also
possible to use Partial Differential Equations (the PDE
approach). The correspondence between the stochastic problem and
its related PDE representation is established by the help of
Feynman-Kac theorem. Also in their original paper, Black and
Scholes transferred the stochastic representation of the problem
into its corresponding PDE, the heat equation. After solving the
heat equation, they transformed the solution back into the
relevant option price. As a third type of methods, one can employ
numerical methods such as Monte Carlo methods.
Monte Carlo methods are especially useful to compute the expected
value of a random variable. Roughly speaking, instead of
examining the probabilistic evolution of this random variable, we
focus on the possible outcomes of it. One generates random
numbers with the same distribution as the random variable and
then we simulate possible outcomes by using those random numbers.
Then we replace the expected value of the random variable by
taking the arithmetic average of the possible outcomes obtained
by the Monte Carlo simulation. The idea of Monte Carlo is simple.
However, it takes its strength from two essential theorems,
namely Kolmogorov’s strong law of large numbers which ensures
convergence of the estimates and the central limit theorem, which
refers to the error distribution of our estimates.
Electricity markets exhibit certain properties which we do not
observe in other markets. Those properties are mainly due to the
unique characteristics of the production and consumption of
electricity. Most importantly one cannot physically store
electricity. This leads to several differences compared to other
financial markets. For example, we observe spikes in electricity
prices. Spikes refer to sudden upward or downward jumps which are
followed by a fast reversion to the mean level. Therefore,
electricity prices show extreme variability compared to other
commodities or stocks.
For example, in stock markets we observe a moderate volatility
level ranging between 1% and 1.5%, commodities like crude oil or
natural gas have relatively high volatilities ranging between
1.5% and 4% and finally the electricity energy has up to 50%
volatility (Weron, 2000). Moreover, electricity prices show
strong seasonality which is related to day to day and month to
month variations in the electricity consumption. In other words,
electricity consumption varies depending on the day of the week
and month of the year. Another important property of the
electricity prices is that they follow a mean reverting process.
Thus, the Ornstein-Uhlenbeck (OU) process which has a Gaussian
distribution is widely used to model electricity prices. In order
to incorporate the spike behavior of the electricity prices, a
jump or a Levy component is merged into the OU process. These
models are known as generalized OU processes (Barndorff-Nielsen
& Shephard, 2001; Benth, Kallsen & Meyer-Brandis, 2007).
There exist several models to capture those properties of
electricity prices. For example, structural models which are
based on the equilibrium of supply and demand (Barlow, 2002),
Markov jump diffusion models which combine the OU process with
pure jump diffusions (Geman & Roncoroni, 2006),
regime-switching models which aim to distinguish the base and
spike regimes of the electricity prices and finally the
multi-factor models which have a deterministic component for
seasonality, a mean reverting process for the base signal and a
jump or Levy process for spikes (Meyer-Brandis & Tankov,
2008).
The German electricity market is one of the largest in Europe.
The energy strategy of Germany follows the objective to phase out
the nuclear power plants by 2021 and gradually introduce
renewable energy ressources. For electricity production, the
share of renewable ressources will increase up to 80% by 2050.
The introduction of renewable ressources brings also some
challenges for electricity trading. For example, the forecast
errors regarding the electricity production might cause high risk
for market participants. However, the developed market structure
of Germany is designed to reduce this risk as much as possible.
There are two main electricity spot price markets where the
market participants can trade electricity. The first one is the
day-ahead market in which the trading takes place around noon on
the day before the delivery. In this market, the trades are based
on auctions. The second one is the intraday market in which the
trading starts at 3pm on the day before the delivery and
continues up until 30 minutes before the delivery. Intraday
market allows continuous trading of electricity which indeed
helps the market participants to adjust their positions more
precisely in the market by reducing the forecast errors.
References
S. Coskun and R. Korn: Pricing Barrier Options in the Heston
Model Using the Heath-Platen estimator. Monte Carlo Methods and
Applications. 24 (1) 29-42, 2018.
S. Coskun: Application of the Heath–Platen Estimator in
Pricing Barrier and Bond Options. PhD thesis, Department of
Mathematics, University of Kaiserslautern, Germany, 2017.
S. Desmettre and R. Korn: 10 Computationally challenging
problems in Finance. FPGA Based Accelerators for Financial
Applications, Springer, Heidelberg, 1–32, 2015.
F. Black and M. Scholes: The pricing of options and corporate
liabilities. The Journal of Political Economy, 81(3):637-654,
1973.
S.L. Heston: A closed-form solution for options with
stochastic volatility with applications to bond and currency
options. The Review of Financial Studies, 6(2):327–343, 1993.
R. Korn, E. Korn and G. Kroisandt: Monte Carlo Methods and
Models in Finance and Insurance. Chapman & Hall/CRC Financ.
Math. Ser., CRC Press, Boca Raton, 2010.
P. Glasserman, Monte Carlo Methods in Financial Engineering.
Stochastic Modelling and Applied Probability, Appl. Math. (New
York) 53, Springer, New York, 2004.
M.T. Barlow: A diffusion model for electricity prices.
Mathematical Finance, 12(4):287-298, 2002.
O.E. Barndorff-Nielsen and N. Shephard: Non-Gaussian
Ornstein-Uhlenbeck-based models and some of their uses in
financial economics. Journal of the Royal Statistical Society B,
63(2):167-241, 2001.
H. Geman and A. Roncoroni: Understanding the fine structure
of electricity prices. The Journal of Business, 79(3):1225-1261,
2006.
T. Meyer-Brandis and P. Tankov: Multi-factor jump-diffusion
models of electricity prices. International Journal of
Theoretical and Applied Finance, 11(5):503-528, 2008.
R. Weron: Energy price risk management. Physica A,
285(1-2):127–134, 2000.
Podcasts
G. Thäter, M. Hofmanová: Turbulence, conversation in the
Modellansatz Podcast, episode 155, Department of Mathematics,
Karlsruhe Institute of Technology (KIT), 2018.
http://modellansatz.de/turbulence
G. Thäter, M. J. Amtenbrink: Wasserstofftankstellen, Gespräch
im Modellansatz Podcast, Folge 163, Fakultät für Mathematik,
Karlsruher Institut für Technologie (KIT), 2018.
http://modellansatz.de/wasserstofftankstellen
S. Ajuvo, S. Ritterbusch: Finanzen damalsTM, Gespräch im
Modellansatz Podcast, Folge 97, Fakultät für Mathematik,
Karlsruher Institut für Technologie (KIT), 2016.
http://modellansatz.de/finanzen-damalstm
K. Cindric, G. Thäter: Kaufverhalten, Gespräch im
Modellansatz Podcast, Folge 45, Fakultät für Mathematik,
Karlsruher Institut für Technologie (KIT), 2015.
http://modellansatz.de/kaufverhalten
V. Riess, G. Thäter: Gasspeicher, Gespräch im Modellansatz
Podcast, Folge 23, Fakultät für Mathematik, Karlsruher Institut
für Technologie (KIT), 2015. http://modellansatz.de/gasspeicher
F. Schueth, T. Pritlove: Energieforschung, Episode 12 im
Forschergeist Podcast, Stifterverband/Metaebene, 2015.
https://forschergeist.de/podcast/fg012-energieforschung/
Weitere Episoden

16 Minuten
vor 2 Monaten

1 Stunde 42 Minuten
vor 7 Monaten

50 Minuten
vor 1 Jahr

42 Minuten
vor 1 Jahr

35 Minuten
vor 1 Jahr
Abonnenten
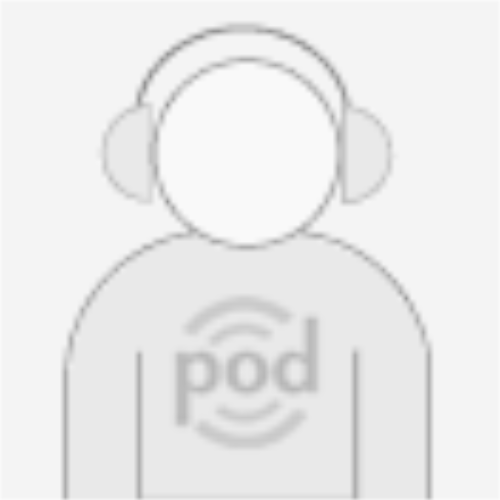
Neutraubing
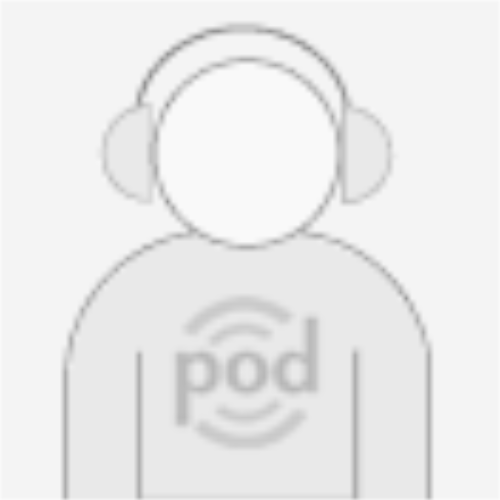
Darmstadt
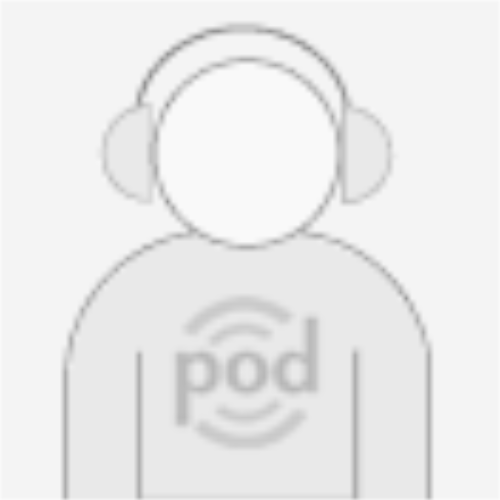
Groß Gusborn
Kommentare (0)