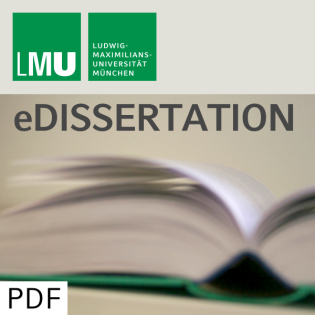
Similarity search and mining in uncertain spatial and spatio-temporal databases
Beschreibung
vor 10 Jahren
Both the current trends in technology such as smart phones, general
mobile devices, stationary sensors and satellites as well as a new
user mentality of utilizing this technology to voluntarily share
information produce a huge flood of geo-spatial and
geo-spatio-temporal data. This data flood provides a tremendous
potential of discovering new and possibly useful knowledge. In
addition to the fact that measurements are imprecise, due to the
physical limitation of the devices, some form of interpolation is
needed in-between discrete time instances. From a complementary
perspective - to reduce the communication and bandwidth
utilization, along with the storage requirements, often the data is
subjected to a reduction, thereby eliminating some of the
known/recorded values. These issues introduce the notion of
uncertainty in the context of spatio-temporal data management - an
aspect raising an imminent need for scalable and flexible data
management. The main scope of this thesis is to develop effective
and efficient techniques for similarity search and data mining in
uncertain spatial and spatio-temporal data. In a plethora of
research fields and industrial applications, these techniques can
substantially improve decision making, minimize risk and unearth
valuable insights that would otherwise remain hidden. The challenge
of effectiveness in uncertain data is to correctly determine the
set of possible results, each associated with the correct
probability of being a result, in order to give a user a confidence
about the returned results. The contrary challenge of efficiency,
is to compute these result and corresponding probabilities in an
efficient manner, allowing for reasonable querying and mining
times, even for large uncertain databases. The paradigm used to
master both challenges, is to identify a small set of equivalent
classes of possible worlds, such that members of the same class can
be treated as equivalent in the context of a given query predicate
or data mining task. In the scope of this work, this paradigm will
be formally defined, and applied to the most prominent classes of
spatial queries on uncertain data, including range queries,
k-nearest neighbor queries, ranking queries and reverse k-nearest
neighbor queries. For this purpose, new spatial and probabilistic
pruning approaches are developed to further speed up query
processing. Furthermore, the proposed paradigm allows to develop
the first efficient solution for the problem of frequent
co-location mining on uncertain data. Special emphasis is taken on
the temporal aspect of applications using modern data collection
technologies. While the aforementioned techniques work well for
single points of time, the prediction of query results over time
remains a challenge. This thesis fills this gap by modeling an
uncertain spatio-temporal object as a stochastic process, and by
applying the above paradigm to efficiently query, index and mine
historical spatio-temporal data.
mobile devices, stationary sensors and satellites as well as a new
user mentality of utilizing this technology to voluntarily share
information produce a huge flood of geo-spatial and
geo-spatio-temporal data. This data flood provides a tremendous
potential of discovering new and possibly useful knowledge. In
addition to the fact that measurements are imprecise, due to the
physical limitation of the devices, some form of interpolation is
needed in-between discrete time instances. From a complementary
perspective - to reduce the communication and bandwidth
utilization, along with the storage requirements, often the data is
subjected to a reduction, thereby eliminating some of the
known/recorded values. These issues introduce the notion of
uncertainty in the context of spatio-temporal data management - an
aspect raising an imminent need for scalable and flexible data
management. The main scope of this thesis is to develop effective
and efficient techniques for similarity search and data mining in
uncertain spatial and spatio-temporal data. In a plethora of
research fields and industrial applications, these techniques can
substantially improve decision making, minimize risk and unearth
valuable insights that would otherwise remain hidden. The challenge
of effectiveness in uncertain data is to correctly determine the
set of possible results, each associated with the correct
probability of being a result, in order to give a user a confidence
about the returned results. The contrary challenge of efficiency,
is to compute these result and corresponding probabilities in an
efficient manner, allowing for reasonable querying and mining
times, even for large uncertain databases. The paradigm used to
master both challenges, is to identify a small set of equivalent
classes of possible worlds, such that members of the same class can
be treated as equivalent in the context of a given query predicate
or data mining task. In the scope of this work, this paradigm will
be formally defined, and applied to the most prominent classes of
spatial queries on uncertain data, including range queries,
k-nearest neighbor queries, ranking queries and reverse k-nearest
neighbor queries. For this purpose, new spatial and probabilistic
pruning approaches are developed to further speed up query
processing. Furthermore, the proposed paradigm allows to develop
the first efficient solution for the problem of frequent
co-location mining on uncertain data. Special emphasis is taken on
the temporal aspect of applications using modern data collection
technologies. While the aforementioned techniques work well for
single points of time, the prediction of query results over time
remains a challenge. This thesis fills this gap by modeling an
uncertain spatio-temporal object as a stochastic process, and by
applying the above paradigm to efficiently query, index and mine
historical spatio-temporal data.
Weitere Episoden

vor 10 Jahren

vor 10 Jahren

vor 10 Jahren

vor 10 Jahren
Kommentare (0)