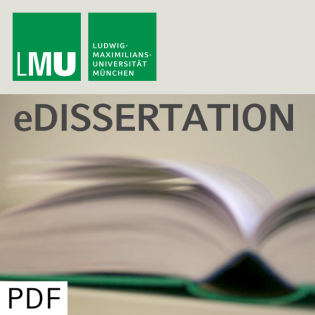
Exploiting prior knowledge and latent variable representations for the statistical modeling and probabilistic querying of large knowledge graphs
Beschreibung
vor 8 Jahren
Large knowledge graphs increasingly add great value to various
applications that require machines to recognize and understand
queries and their semantics, as in search or question answering
systems. These applications include Google search, Bing search,
IBM’s Watson, but also smart mobile assistants as Apple’s Siri,
Google Now or Microsoft’s Cortana. Popular knowledge graphs like
DBpedia, YAGO or Freebase store a broad range of facts about the
world, to a large extent derived from Wikipedia, currently the
biggest web encyclopedia. In addition to these freely accessible
open knowledge graphs, commercial ones have also evolved including
the well-known Google Knowledge Graph or Microsoft’s Satori. Since
incompleteness and veracity of knowledge graphs are known problems,
the statistical modeling of knowledge graphs has increasingly
gained attention in recent years. Some of the leading approaches
are based on latent variable models which show both excellent
predictive performance and scalability. Latent variable models
learn embedding representations of domain entities and relations
(representation learning). From these embeddings, priors for every
possible fact in the knowledge graph are generated which can be
exploited for data cleansing, completion or as prior knowledge to
support triple extraction from unstructured textual data as
successfully demonstrated by Google’s Knowledge-Vault project.
However, large knowledge graphs impose constraints on the
complexity of the latent embeddings learned by these models. For
graphs with millions of entities and thousands of relation-types,
latent variable models are required to exploit low dimensional
embeddings for entities and relation-types to be tractable when
applied to these graphs. The work described in this thesis extends
the application of latent variable models for large knowledge
graphs in three important dimensions. First, it is shown how the
integration of ontological constraints on the domain and range of
relation-types enables latent variable models to exploit latent
embeddings of reduced complexity for modeling large knowledge
graphs. The integration of this prior knowledge into the models
leads to a substantial increase both in predictive performance and
scalability with improvements of up to 77% in link-prediction
tasks. Since manually designed domain and range constraints can be
absent or fuzzy, we also propose and study an alternative approach
based on a local closed-world assumption, which derives domain and
range constraints from observed data without the need of prior
knowledge extracted from the curated schema of the knowledge graph.
We show that such an approach also leads to similar significant
improvements in modeling quality. Further, we demonstrate that
these two types of domain and range constraints are of general
value to latent variable models by integrating and evaluating them
on the current state of the art of latent variable models
represented by RESCAL, Translational Embedding, and the neural
network approach used by the recently proposed Google Knowledge
Vault system. In the second part of the thesis it is shown that the
just mentioned three approaches all perform well, but do not share
many commonalities in the way they model knowledge graphs. These
differences can be exploited in ensemble solutions which improve
the predictive performance even further. The third part of the
thesis concerns the efficient querying of the statistically modeled
knowledge graphs. This thesis interprets statistically modeled
knowledge graphs as probabilistic databases, where the latent
variable models define a probability distribution for triples. From
this perspective, link-prediction is equivalent to querying ground
triples which is a standard functionality of the latent variable
models. For more complex querying that involves e.g. joins and
projections, the theory on probabilistic databases provides
evaluation rules. In this thesis it is shown how the intrinsic
features of latent variable models can be combined with the theory
of probabilistic databases to realize efficient probabilistic
querying of the modeled graphs.
applications that require machines to recognize and understand
queries and their semantics, as in search or question answering
systems. These applications include Google search, Bing search,
IBM’s Watson, but also smart mobile assistants as Apple’s Siri,
Google Now or Microsoft’s Cortana. Popular knowledge graphs like
DBpedia, YAGO or Freebase store a broad range of facts about the
world, to a large extent derived from Wikipedia, currently the
biggest web encyclopedia. In addition to these freely accessible
open knowledge graphs, commercial ones have also evolved including
the well-known Google Knowledge Graph or Microsoft’s Satori. Since
incompleteness and veracity of knowledge graphs are known problems,
the statistical modeling of knowledge graphs has increasingly
gained attention in recent years. Some of the leading approaches
are based on latent variable models which show both excellent
predictive performance and scalability. Latent variable models
learn embedding representations of domain entities and relations
(representation learning). From these embeddings, priors for every
possible fact in the knowledge graph are generated which can be
exploited for data cleansing, completion or as prior knowledge to
support triple extraction from unstructured textual data as
successfully demonstrated by Google’s Knowledge-Vault project.
However, large knowledge graphs impose constraints on the
complexity of the latent embeddings learned by these models. For
graphs with millions of entities and thousands of relation-types,
latent variable models are required to exploit low dimensional
embeddings for entities and relation-types to be tractable when
applied to these graphs. The work described in this thesis extends
the application of latent variable models for large knowledge
graphs in three important dimensions. First, it is shown how the
integration of ontological constraints on the domain and range of
relation-types enables latent variable models to exploit latent
embeddings of reduced complexity for modeling large knowledge
graphs. The integration of this prior knowledge into the models
leads to a substantial increase both in predictive performance and
scalability with improvements of up to 77% in link-prediction
tasks. Since manually designed domain and range constraints can be
absent or fuzzy, we also propose and study an alternative approach
based on a local closed-world assumption, which derives domain and
range constraints from observed data without the need of prior
knowledge extracted from the curated schema of the knowledge graph.
We show that such an approach also leads to similar significant
improvements in modeling quality. Further, we demonstrate that
these two types of domain and range constraints are of general
value to latent variable models by integrating and evaluating them
on the current state of the art of latent variable models
represented by RESCAL, Translational Embedding, and the neural
network approach used by the recently proposed Google Knowledge
Vault system. In the second part of the thesis it is shown that the
just mentioned three approaches all perform well, but do not share
many commonalities in the way they model knowledge graphs. These
differences can be exploited in ensemble solutions which improve
the predictive performance even further. The third part of the
thesis concerns the efficient querying of the statistically modeled
knowledge graphs. This thesis interprets statistically modeled
knowledge graphs as probabilistic databases, where the latent
variable models define a probability distribution for triples. From
this perspective, link-prediction is equivalent to querying ground
triples which is a standard functionality of the latent variable
models. For more complex querying that involves e.g. joins and
projections, the theory on probabilistic databases provides
evaluation rules. In this thesis it is shown how the intrinsic
features of latent variable models can be combined with the theory
of probabilistic databases to realize efficient probabilistic
querying of the modeled graphs.
Weitere Episoden

vor 8 Jahren

vor 8 Jahren
Kommentare (0)