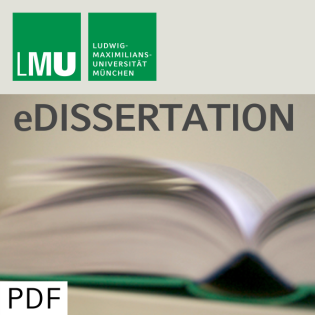
Network-based analysis of gene expression data
Beschreibung
vor 8 Jahren
The methods of molecular biology for the quantitative measurement
of gene expression have undergone a rapid development in the past
two decades. High-throughput assays with the microarray and RNA-seq
technology now enable whole-genome studies in which several
thousands of genes can be measured at a time. However, this has
also imposed serious challenges on data storage and analysis, which
are subject of the young, but rapidly developing field of
computational biology. To explain observations made on such a large
scale requires suitable and accordingly scaled models of gene
regulation. Detailed models, as available for single genes, need to
be extended and assembled in larger networks of regulatory
interactions between genes and gene products. Incorporation of such
networks into methods for data analysis is crucial to identify
molecular mechanisms that are drivers of the observed expression.
As methods for this purpose emerge in parallel to each other and
without knowing the standard of truth, results need to be
critically checked in a competitive setup and in the context of the
available rich literature corpus. This work is centered on and
contributes to the following subjects, each of which represents
important and distinct research topics in the field of
computational biology: (i) construction of realistic gene
regulatory network models; (ii) detection of subnetworks that are
significantly altered in the data under investigation; and (iii)
systematic biological interpretation of detected subnetworks. For
the construction of regulatory networks, I review existing methods
with a focus on curation and inference approaches. I first describe
how literature curation can be used to construct a regulatory
network for a specific process, using the well-studied diauxic
shift in yeast as an example. In particular, I address the question
how a detailed understanding, as available for the regulation of
single genes, can be scaled-up to the level of larger systems. I
subsequently inspect methods for large-scale network inference
showing that they are significantly skewed towards master
regulators. A recalibration strategy is introduced and applied,
yielding an improved genome-wide regulatory network for yeast. To
detect significantly altered subnetworks, I introduce GGEA as a
method for network-based enrichment analysis. The key idea is to
score regulatory interactions within functional gene sets for
consistency with the observed expression. Compared to other
recently published methods, GGEA yields results that consistently
and coherently align expression changes with known regulation types
and that are thus easier to explain. I also suggest and discuss
several significant enhancements to the original method that are
improving its applicability, outcome and runtime. For the
systematic detection and interpretation of subnetworks, I have
developed the EnrichmentBrowser software package. It implements
several state-of-the-art methods besides GGEA, and allows to
combine and explore results across methods. As part of the
Bioconductor repository, the package provides a unified access to
the different methods and, thus, greatly simplifies the usage for
biologists. Extensions to this framework, that support automating
of biological interpretation routines, are also presented. In
conclusion, this work contributes substantially to the research
field of network-based analysis of gene expression data with
respect to regulatory network construction, subnetwork detection,
and their biological interpretation. This also includes recent
developments as well as areas of ongoing research, which are
discussed in the context of current and future questions arising
from the new generation of genomic data.
of gene expression have undergone a rapid development in the past
two decades. High-throughput assays with the microarray and RNA-seq
technology now enable whole-genome studies in which several
thousands of genes can be measured at a time. However, this has
also imposed serious challenges on data storage and analysis, which
are subject of the young, but rapidly developing field of
computational biology. To explain observations made on such a large
scale requires suitable and accordingly scaled models of gene
regulation. Detailed models, as available for single genes, need to
be extended and assembled in larger networks of regulatory
interactions between genes and gene products. Incorporation of such
networks into methods for data analysis is crucial to identify
molecular mechanisms that are drivers of the observed expression.
As methods for this purpose emerge in parallel to each other and
without knowing the standard of truth, results need to be
critically checked in a competitive setup and in the context of the
available rich literature corpus. This work is centered on and
contributes to the following subjects, each of which represents
important and distinct research topics in the field of
computational biology: (i) construction of realistic gene
regulatory network models; (ii) detection of subnetworks that are
significantly altered in the data under investigation; and (iii)
systematic biological interpretation of detected subnetworks. For
the construction of regulatory networks, I review existing methods
with a focus on curation and inference approaches. I first describe
how literature curation can be used to construct a regulatory
network for a specific process, using the well-studied diauxic
shift in yeast as an example. In particular, I address the question
how a detailed understanding, as available for the regulation of
single genes, can be scaled-up to the level of larger systems. I
subsequently inspect methods for large-scale network inference
showing that they are significantly skewed towards master
regulators. A recalibration strategy is introduced and applied,
yielding an improved genome-wide regulatory network for yeast. To
detect significantly altered subnetworks, I introduce GGEA as a
method for network-based enrichment analysis. The key idea is to
score regulatory interactions within functional gene sets for
consistency with the observed expression. Compared to other
recently published methods, GGEA yields results that consistently
and coherently align expression changes with known regulation types
and that are thus easier to explain. I also suggest and discuss
several significant enhancements to the original method that are
improving its applicability, outcome and runtime. For the
systematic detection and interpretation of subnetworks, I have
developed the EnrichmentBrowser software package. It implements
several state-of-the-art methods besides GGEA, and allows to
combine and explore results across methods. As part of the
Bioconductor repository, the package provides a unified access to
the different methods and, thus, greatly simplifies the usage for
biologists. Extensions to this framework, that support automating
of biological interpretation routines, are also presented. In
conclusion, this work contributes substantially to the research
field of network-based analysis of gene expression data with
respect to regulatory network construction, subnetwork detection,
and their biological interpretation. This also includes recent
developments as well as areas of ongoing research, which are
discussed in the context of current and future questions arising
from the new generation of genomic data.
Weitere Episoden

vor 8 Jahren

vor 8 Jahren
Kommentare (0)